
Improving Access to Testing in Remote Areas with Machine Learning
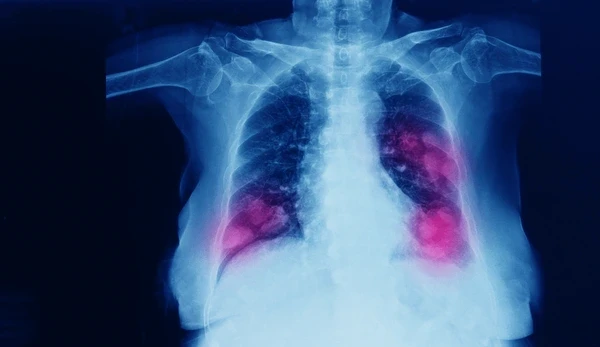
The problem
In a lot of remote rural parts of India, Africa and Latin America, residents often have next to zero access to any medical care at all; be it doctors or even radiologists, let alone specialists. But that doesn’t stop them falling sick. In the event that someone does fall sick and is in need of medical attention, they are usually taken to a hospital or clinic in the nearest city which could often be hours away. The situation becomes even more bleak when it comes to access to diagnostics, imaging or specialists.
How could machine learning help?
Automated diagnoses through machine learning models could be used to bridge this gap. This use case focuses on how machine learning could improve access to testing in remote areas with mobile healthcare initiative. This would involve the deployment of Mobile Diagnostic Units(MDUs), special vehicles equipped with medical imaging devices, portable diagnostic equipment and connectivity tools that can reach remote communities
Workflow:
Deployment :-
The MDU is dispatched to remote areas, reaching communities that lack proximity to fixed healthcare infrastructure.
Data collection :-
Patients undergo diagnostic tests - including imaging scans, blood tests and other relevant examinations within the mobile unit.
The collected data is processed locally using machine learning algorithms embedded in the mobile diagnostic system.
Real-time analysis :-
Machine learning algorithms for - image recognition and pathology detection, analyze medical images and data in real-time to identify potential health conditions or abnormalities.
Telemedicine consultation :-
The results(along with patient information) are transmitted securely to a central medical hub.
Healthcare professionals at the central hub use telemedicine platforms to remotely consult with patients, providing timely feedback and recommendations.
Customized care plans :-
Based on the diagnostic results, personalized care plans and recommendations are generated with the assistance of machine learning algorithms.
The mobile unit is equipped to dispense basic medications or provide immediate interventions for certain conditions.
Benefits :-
Improved accessibility :-
Enables healthcare access in remote and underserved areas where traditional medical facilities are scarce.
Early detection and intervention :-
Machine learning algorithms aid in the early detection of health issues, allowing for prompt intervention and treatment.
Cost-effective healthcare :-
Reduces the need for patients to travel long distances for diagnostics i.e. making healthcare more cost-effective for both patients and healthcare providers.
Enhanced efficiency :-
Streamlines the diagnostic process with automated analysis i.e. optimizing the use of healthcare resources.
Data-driven decision making :-
The collected data contributes to population health studies, informing public health policies and resource allocation.
Where data annotation comes in :-
The most crucial part of this entire process are the trained machine learning models for image recognition, pathology detection and diagnostic analysis(e.g. detect tumors in x-rays). These models, that are capable of identifying potential health issues, need to be trained on vast amounts of data. Data that has to first be annotated by human annotators; specifically, experienced radiologists. The performance of these models depends heavily on vast amounts of accurately labeled training data. It could involve various kinds of annotations - be it image segmentation, image classification, keypoint annotation and so on, by experts in the field.