
Enhancing Legal Language Models (LLMs) through Human Feedback
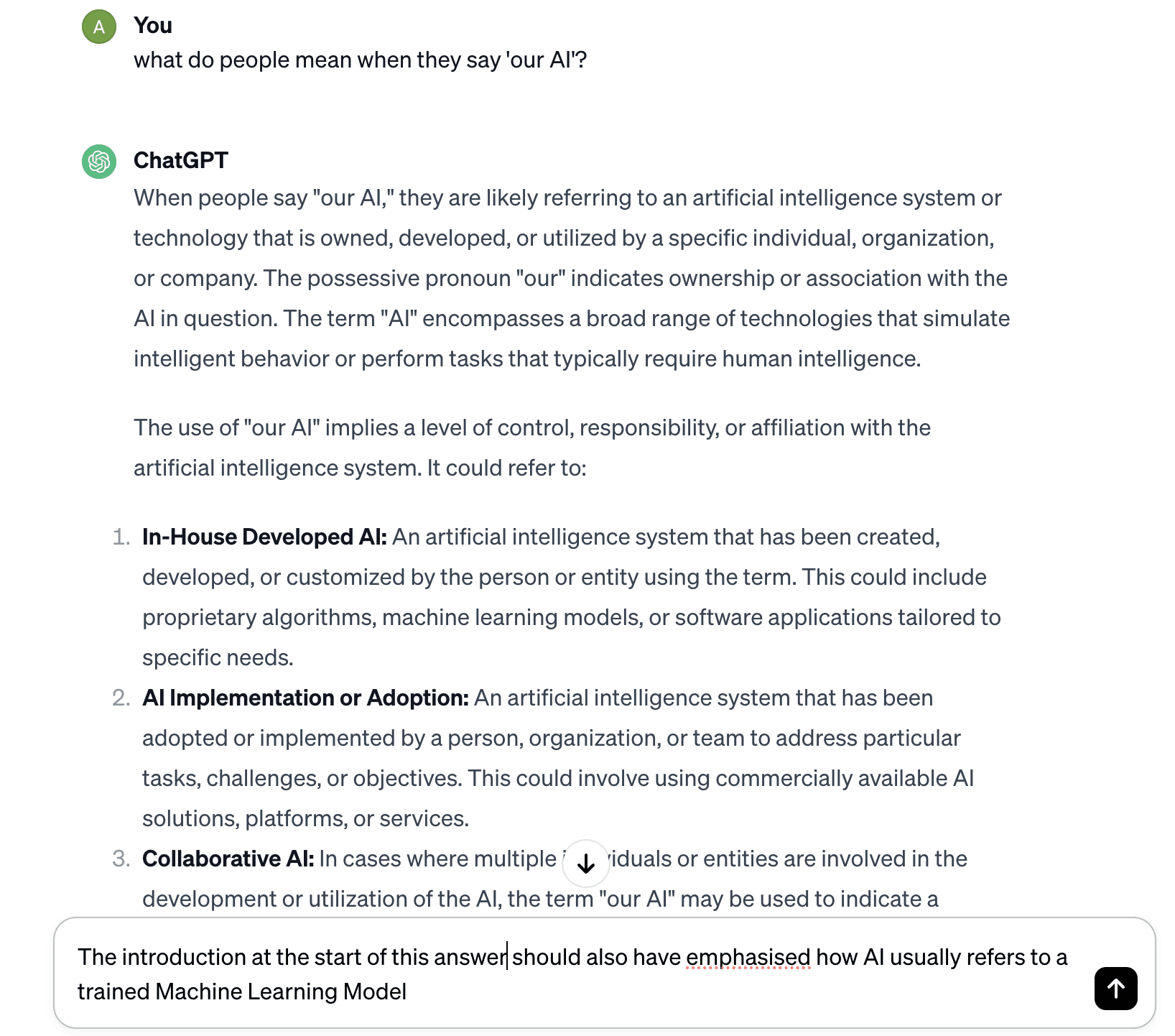
Legal Language Models, have become invaluable tools in the legal field for tasks such as legal research, contract analysis, and document summarization. While LLMs exhibit impressive capabilities, the dynamic and nuanced nature of legal language demands continuous improvement. Human feedback plays a crucial role in refining LLMs to ensure accuracy, relevance, and ethical considerations.
Where Human Feedback is Needed:
Legal Expertise:
Human feedback from legal professionals, including attorneys, paralegals, and legal researchers, provides valuable insights into the nuances of legal language, contextual relevance, and evolving legal practices.
Ethical Considerations:
Legal systems often involve ethical considerations and cultural context. Human feedback helps in refining LLMs to align with ethical guidelines, avoiding biases, and considering cultural sensitivities.
Ambiguity Resolution:
Legal documents often contain ambiguity and complex language structures. Human feedback assists in resolving ambiguities, clarifying context, and ensuring that LLMs interpret legal text accurately.
New Legal Precedents:
Legal systems evolve with new precedents, case laws, and legislative changes. Human experts can provide real-time feedback to update LLMs and ensure they reflect the latest legal developments.
User-specific Adaptations:
Legal professionals often require tailored solutions for specific practices or jurisdictions. Human feedback helps customize LLMs to meet the unique needs of legal practitioners in different contexts.
Workflow:
Data Annotation:
Legal professionals review and annotate datasets used for training LLMs, marking instances where the model's understanding may deviate from legal norms.
Feedback Loops:
Continuous feedback loops are established where legal practitioners review LLM-generated outputs, providing feedback on both accurate and inaccurate results.
Ambiguity Resolution Sessions:
Periodic sessions involve legal experts and AI developers to address ambiguous scenarios, enhancing the model's ability to handle intricacies within legal language.
Case Simulation:
Simulated legal cases are presented to the LLM, and human experts assess the model's responses, correcting any misinterpretations or oversights.
User Feedback Integration:
End-users, such as law firms or legal departments, contribute feedback on LLM performance in real-world scenarios, helping to fine-tune the model for practical applications.
Benefits:
Accuracy and Reliability:
Human feedback ensures that LLMs accurately interpret legal language, reducing the risk of misinterpretations and inaccuracies in legal analysis.
Adaptability to Legal Changes:
Rapid incorporation of new legal precedents and legislative changes ensures that LLMs stay up-to-date and relevant in a dynamic legal landscape.
Ethical Compliance:
Human feedback aids in identifying and rectifying biases, promoting ethical considerations, and aligning LLMs with legal and cultural norms.
Tailored Solutions:
Customization based on user feedback allows LLMs to adapt to specific legal practices, jurisdictions, and user requirements, enhancing their practical utility.
Enhanced User Confidence:
Legal professionals can trust LLMs as reliable tools when they actively contribute to the improvement process, fostering user confidence and acceptance.
In conclusion, the incorporation of human feedback into the development and refinement of Legal Language Models ensures that these AI systems not only align with legal expertise but also stay responsive to evolving legal landscapes and user needs. Regular Human Feedback becomes crucial to optimize LLMs for real-world legal applications.